Can we save AI? A philosophical answer
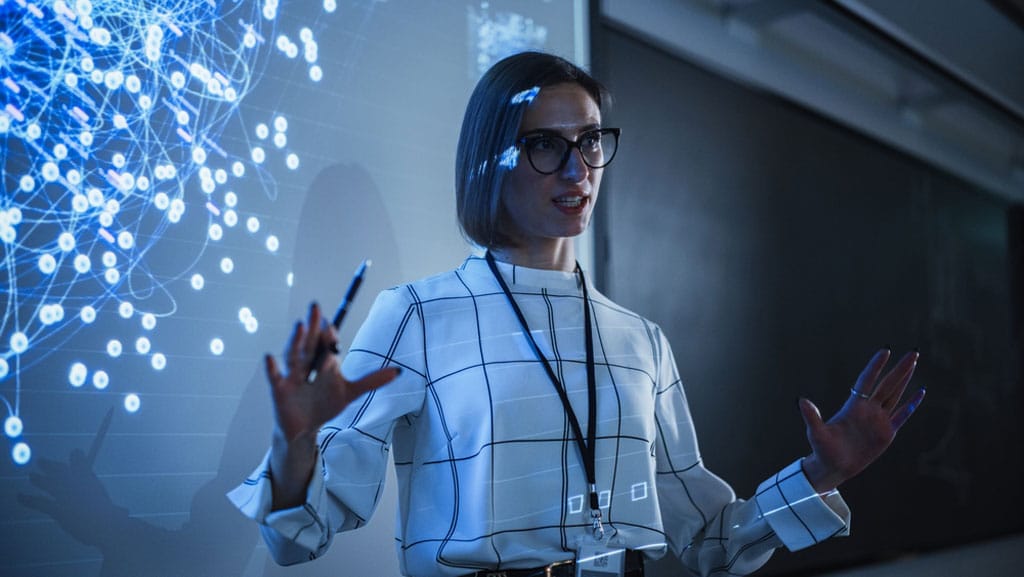
What is knowledge? Why should we bother with this as we build the next generation of artificial intelligence models to replace and outperform humans in all intellectual tasks and decisions?
This is a fundamental question, and it’s a case of always having the right people (read, humans) in the room as we shape the tech that will run the world. Some of these people are the philosophers who used to be at the AI party when the music started. Something happened along the way, which sent them away and lowered their influence. If the tech industry is moving towards the goal of Artificial General Intelligence (AGI,) as stated by its big players, we should make sure that the engineering, funding, and fast deployment of new tools (the priority right now) are helped by the awareness of what machines know and should know, before they produce an output or after that. Why is this relevant? Let’s save AI: Let’s make it as similar to us as possible. It has been its goal since the 1950s anyway.
The original alliance: functionalism
When John McCarthy coined the term “Artificial Intelligence” at the Dartmouth Conference in 1956, he described his intention in clear terms: “Make a machine behave in ways that would be considered intelligent as if a human being was doing it.” McCarthy and his colleagues wanted to create machines that could use language, form abstractions, solve complex problems and even improve themselves independently.
During the first seven decades of its existence, AI has achieved remarkable success, but the holy grail of general intelligence (“make computers do the sorts of things that minds do,” to use Boden’s definition) was not found. Yet, the stated goal of the AI leaders raises a key epistemological question: can machines create knowledge from experience in ways that are like human cognition? That is, “whatever we (humans) possess, whether we call it intelligence, thought, cognition, mind or whatever, can machines possess it too?”
Of course, humans are physically different from machines, as we have biological bodies and brains made of cells. The ambition of AI rests on the idea that intelligence is not about the hardware but about the functional processes that hardware unlocks. What matters is not the machine per se but the virtual machine, which is an information processing system extrinsic to its physical substrate. Therefore, and not surprisingly, AI was a loyal ally to a movement called functionalism, a philosophy of the mind popularized by scholars such as Putnam, Block, and Fodor in the 1960s and 1970s.
According to functionalism, cognition does not depend on the substrate it uses to compute but on the operations performed via its infrastructure. The human mind and a computer can, consequently, share the same kind of “intelligence” if both perform similar functions, regardless of where they are instantiated, neurons or circuits. Based on this principle, classical cognitivism sees cognition as a series of symbolic operations that process information to generate responses. The idea is simple and intuitive: cognitive processes are computations which leverage experience (input) to produce coherent internal representations, which in turn become the basis for further processing up to an eventual action (output). To use an example by David Marr, when we look at a cup of coffee, the brain processes visual stimuli, until it creates a three-dimensional representation of the object, which can then prompt actions, such as the motion of grasping it.
The functionalist view, which dominated AI research in the 1960s and 1970s, failed to solve the question of a general intelligence. Advanced systems were developed, for specific tasks (from speech recognition to playing chess), but the ultimate prize, a super-intelligence, which can adapt and learn like a human, or better, never came to life.
Modern AI: neural networks and GPUs
AI moved away from functionalism, shifting its focus from a hardware-independent cognitivism to a new vision, centered around the materiality of computation. Deep learning, the AI revolution brought to the world by Hinton in the 1980s, replicates the functioning of our brain, and, to do so, it requires enormous computational resources and specialized hardware, such as Graphics Processing Units (GPUs,) which are essential to train large-scale neural networks.
Today’s AI heavily relies on hardware configurations, massive energy requirements and increasing capital investments. Its symbolic architecture, to use the functionalist jargon, is peripheral to any progress on the use cases that it needs to deliver, to please businesses and shareholders. Dependence on GPUs marks a move towards a materialized functionalism, where intelligence depends as much on physical resources as on functional processes. Modern AI needs engineering, before anything else. Intelligence has lost abstraction, becoming the physical gear itself.
Should we worry? The epistemological question is still there: can hardware-constrained systems replicate human cognition, or are they a superficial approximation, for now, because they are conditioned by material constraints? When limitations are overcome, can machines independently find an answer to McCarthy’s original dilemma? Do they know what they know? Is that relevant to us?
Embodiment and interaction: the nouvelle AI.
In the 1980s, the so-called nouvelle AI movement also criticized functionalism, arguing that cognition is inseparable from the physical context. Rodney Brooks and other researchers8 suggested that cognition arises from the interaction with the environment, and not from mere symbolic computations. What is defined as “embodiment” holds that knowledge is the result of a series of situated actions: to perceive a cup of coffee, to use the previous example, the body must perform physical movements, which are an integral part of the cognitive process. Embodiment challenges the “substrate neutrality” of functionalism. Intelligence cannot be “disembodied.” It is rooted in the physical structure of a machine. Nouvelle AI robots, designed to adapt dynamically to the environment and its obstacles, represent the true “practical intelligence,” which interacts with and serves the world. This perspective, influenced by James Gibson’s ecological psychology9 and Francisco Varela’s autopoiesis, suggests that some forms of knowledge cannot be simulated in the absence of a body. Intelligence stems from the causal functions of cognition and the unique material features of the machine. This view emphasizes how cognitive capabilities are inseparable from the interaction between agent and environment. Perceiving a cup of coffee requires eyes, head and body, but the perception itself is crafted by the sensorial possibilities dictated by the context (grasping the cup is one of the options that the environment gives the observer).
The brain as a predictive machine
The nouvelle AI never became mainstream, because creating fully autonomous robots is difficult. The success of machine learning, and in particular neural networks, found a new ally in cognitive scientists like Andy Clark, who crafted a theory that went even further in stressing the interdependence of the functional and material aspects of cognition. What this means is that cognition becomes a predictive process. According to Clark, the human brain functions as a predictive machine, continuously creating hypotheses and comparing them with real life experiences. This replaces a linear processing model with a continuous feedback and update loop, in which predictions are constantly corrected.
To return to our example, perceiving a cup of coffee is shaped by an underlying brain processing, which feeds a set of likely expectations about sensory inputs and causes. These expectations are constantly compared with sensory information, generating prediction errors (deviations) that are processed through the brain. The traditional view of the brain using sensory data to build a representation of the world is turned upside down. Predictive processing states that the brain is a “prediction machine” that continuously generates hypotheses about the world and compares them with sensory inputs, updating the most likely best-case scenario, by continuously reducing errors. In this architecture, the system consists of a hierarchical, generative model, which actively produces internal representations of the world, based on constant integrations of bottom-up (environment) and top-down information (its own predictions). It’s a loop, where discrepancies between predictions and perceived reality (forecast errors) are used to update and improve a model of the world.
World models and simulations
More recently, and as a further step-up, Yann LeCun proposed a view known as “world models.” An intelligent system should build multiple internal representations of the world, to simulate scenarios and make decisions, even in the absence of direct sensory inputs. LeCun questions the central role of prediction errors as the main mechanism for learning and updating predictions. Artificial intelligence should, rather, learn and plan using internal models that simulate the interaction with the environment, without relying solely on real world feedback. The world model is trained to become a simulator of the environment, which “projects” results of future actions and scenarios. This allows it to anticipate the effects of actions and plan more efficiently, without the need for an error-based, constant feedback from the outside. The system learns from simulated experiences and performs informed actions without a confrontation with sensory inputs. The model transcends modern materialist epistemology and embodiment, to make intelligence and knowledge go beyond the environment, and identifies them with simulation machines that anticipate and fire-proof a palette of most likely experiences.
For LeCun, intelligence does not depend on symbolic sequences (neural networks operate at the sub-symbolic level), but on simulated interactions, made by the material substrate of machines.
SOS to all philosophers!
John McCarthy went often back to philosophy. Although “philosophers have not really come to an agreement [regarding the definition of intelligence] in 2,500 years,” he was convinced, during his whole life, that philosophy could serve well AI.
We need to tackle “the” fundamental question, which is an epistemological one: what does it mean for a machine to “know” something, if it has no consciousness or intentionality? Without consciousness filtering experience, “knowledge” is reduced to a bunch of statistical patterns, devoid of a purpose that can comprehend the world, partially and holistically at the same time.
According to the more classical view, human knowledge is intentional and subjective, while machines lack this active, engaged connection with the world. This introduces an essential epistemic distance: while human knowledge is causal and deductive, AI’s knowledge is correlative, and statistics based. Is AI endowed with knowledge or, just, “meaningful” outputs? We truly need philosophy and epistemology here. What does it mean for machines to “know” something, if they have no direct awareness of the outer world?
According to classical philosophy, knowledge is a relationship between subject and object, mediated by the intentionality of consciousness. This goes back to Brentano and, in phenomenology, to Husserl, before being developed by many other of their followers. Machines lack this intentionality. They do not have consciousness, which structures experiences, to develop an internal point of view, subjective and purely human. Their output often appears as meaningful to our eyes, of course, but does it have true meaning, namely that human thrust to decode reality?
The epistemology of AI raises doubts. The fundamental one being: if knowledge of AI’s large language models (LLMs) is limited to statistical patterns extracted from vast amounts of data, can we say they own knowledge? For many experts, the answer is “undoubtedly not, they are just stochastic parrots,” it is we, humans, and only we who can attribute a meaning to life’s events. Understanding is only metaphorical for LLMs. What’s at stake here? Our future. Machines are not aware of what they create. If so, how can we trust their statistical knowledge? What’s their purpose, if they do not have one?
Conclusions: back to Kant? Back to philosophy’s centrality
One of philosophers’ adages is “let’s go back to Kant.” Maybe Kant, who distinguishes between a sensible knowledge, rooted in experience, and an a priori conceptual structure that shapes that experience, could turn out to be helpful. Modern AI does not have native categories of causality, unity or identity that read the world autonomously, as humans can do. Machine learning is inductive and correlative; human knowledge is deductive and causal. This is a significant epistemological detachment. AI lacks the ability to understand, or to develop a meaning, independently of training data.
There is another philosophical issue that deserves our attention, as it concerns the nature of AI’s “knowledge by correlation” (as opposed to humans’ causation.) Judea Pearl, as the best exemplification of this issue, talks about ladders of causation. Recognition of regularities in observations is the first ladder (I see some smoke from the chimney); action is the second ladder (I am the one who lit the chimney, which produces the smoke); hypothesizing is the third one (what would have happened if I had not lit the chimney?). As for the state of the art, a deep neural network can reach the first step only. Without the other two levels, it has no way of knowing why one pattern answers a question or solves a problem. It only knows that certain combinations of inputs tend to produce outputs that would fit similar situations. This type of “observational” knowledge bears a superficial resemblance to human knowledge, as it remains devoid of the conceptual organization that makes humans move beyond what’s in front of them to develop inferences and theories.
If we are willing to accept that AI, even in its most advanced applications, remains bound to some knowledge without intentionality or understanding of causality, it is mandatory to ask: what are the limits of AI’s epistemic capabilities? Without a Kantian “transcendental structure,” how can machines access autonomous and creative knowledge?
This is philosophical talk. Philosophy cannot only cover ethical or social externalities of AI, or some metaphysical folklore (are machines sentient?) It needs to be at the heart of the discipline. Philosophers and engineers must be in the same room, and rethink together knowledge and understanding of a world increasingly efficient and effective, thanks to automation.
Have you read?
Best Medical Schools In The World.
Best Business Schools In The World.
World’s Best International High Schools.
Best Hospitality And Hotel Management Schools In The World.
World’s Most Powerful Passports.
Bring the best of the CEOWORLD magazine's global journalism to audiences in the United States and around the world. - Add CEOWORLD magazine to your Google News feed.
Follow CEOWORLD magazine headlines on: Google News, LinkedIn, Twitter, and Facebook.
Copyright 2025 The CEOWORLD magazine. All rights reserved. This material (and any extract from it) must not be copied, redistributed or placed on any website, without CEOWORLD magazine' prior written consent. For media queries, please contact: info@ceoworld.biz