Munjal Shah Thinks Hippocratic AI Can Help Solve a Health Care Staffing Crisis with Superstaffing
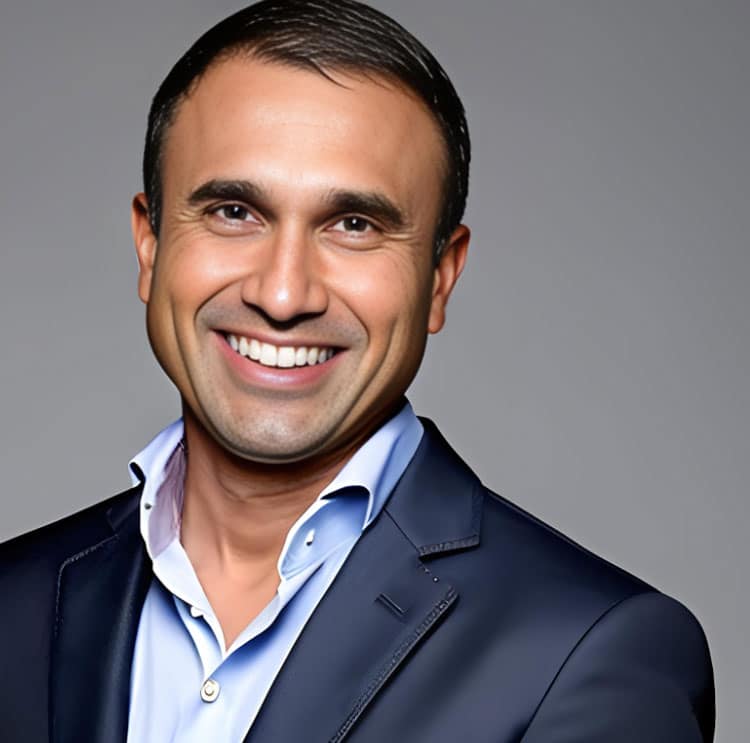
America is in the midst of a crisis in health care staffing levels. In February 2023, the Department of Health and Human Services reported that 16.7% of reporting hospitals anticipated a critical staffing shortage. The Bureau of Labor Statistics recently estimated that U.S. health care organizations will need to fill over 200,000 nursing positions every year until 2031. In a survey conducted during the pandemic in 2021, 30% of nurses said they were contemplating leaving the profession. A 2023 survey found that percentage largely unchanged at 28.7%.
How do we solve this crisis? For Munjal Shah, the founder and CEO of Hippocratic AI, the answer is clear: large language models. Shah thinks that LLMs — the technology behind the explosion of popular chatbots like OpenAI’s ChatGPT and Google’s Bard — can be used to develop effective, inexpensive, and low-risk artificial intelligence for nondiagnostic health care applications and that the downstream effects could drastically improve patient outcomes, lower costs, and increase access to care.
The goal is to use AI to implement what Shah calls “superstaffing.” Superstaffing isn’t just about using AI to fill existing staffing gaps. It is also about enabling new interventions by decreasing the costs of nondiagnostic services.
“Productivity tools can give us maybe 10% to 20% more capacity, but superstaffing can give us 10 times or 100 times more capacity,” says Shah.
“After the pandemic, everybody quit because they got burnt out. This happened. This is happening. And so we basically said, ‘Why don’t we use generative AI for the thing it does best, which is conversation?’” he adds.
“Let it talk to patients and let it be the chronic care nurse. Chronic care nurses don’t diagnose you. You’ve already been diagnosed. They just ask questions like: ‘Did you take your meds? Do you need a ride to your next appointment? Did you make your next appointment? Do you have enough food? Should I call the food bank and see if they can bring you some food tonight, ma’am?’
“And we said, ‘Wow, we don’t do that. We don’t have enough staff to do that. We don’t call patients every day after they’re discharged from the hospital to check in on them. We don’t even call them once in most hospital systems.’”
How Can AI ‘Do No Harm’?
Anyone who has used or even heard of generative AI applications like ChatGPT and Bard has likely also heard of the “hallucination problem.” Put simply, these systems, which are designed to accurately predict, then produce, appropriate text responses to users’ questions or commands, are not always accurate. They “hallucinate,” generating false information and displaying it to the user as fact.
There are plenty of absurd but innocuous examples of this. For instance, when cognitive scientist Douglas Hofstadter asked ChatGPT, “When was the Golden Gate Bridge transported for the second time across Egypt?” it responded, “The Golden Gate Bridge was transported for the second time across Egypt in October of 2016.”
On the other hand, these systems are also capable of jaw-droppingly accurate performances on tasks previously thought to be exclusively the territory of human reason. They’ve passed the bar exam and numerous medical exams. They’ve matched the ability of young children to predict the beliefs and emotions of human beings. And LLMs continue to improve. The hallucination Hofstadter induced was, in fact, made by an earlier model, GPT-3, not the current GPT-4.
For Munjal Shah, who studied medical applications of AI and went on to found several successful AI-based companies, the question was: How can we use the unprecedented abilities of LLMs in the health care space while avoiding the risks of relying on systems that are always, in theory, capable of hallucinating? He thought the lifesaving potential of this leap in predictive AI technology was clear, but agreed that humans should understandably be wary of trusting these systems to give them advice that could harm or kill them if incorrect.
This led to the founding of Hippocratic AI, a company that will focus on low-risk, nondiagnostic applications of LLMs such as chronic care nursing, diet and nutrition counseling, and patient navigation. The company’s name comes from the Hippocratic oath, the essence of which is “do no harm.” Its goal is to help patients using powerful LLMs while avoiding high-risk applications that require diagnostic trust. For Shah, there’s enough to be gained without trying to force this issue.
He believes the way to make AI trustworthy is to pick the right applications that are safer, that have lower risk. “I have no interest in trying to solve the diagnosis. I think it’s too high-risk,” he says.
“We thought, what if we can build a fully automated [information system] that calls people on the phone and talks to them and does preoperative calls before a colonoscopy to make sure you’re taking the drink, that checks in with you to make sure you’re getting that MRI you were supposed to get done but keep blowing off. These are low-risk, actually, but they make a big difference in health care.”
How AI Could Solve the Staffing Crisis
The problem, as Shah sees it, is that, despite the benefits of these low-risk interventions, we simply lack the staff to make them in any consistent way. There aren’t enough nurses to connect with the patients who could benefit from continuing care, not enough dietitians to provide the sort of on-call support someone with a dietary restriction might need, and not enough patient navigators to help every patient find the best facilities and specialists to get the care they need.
But LLMs can provide these services, and the fact that they’re not human helps them avoid burnout. The cost of an LLM, at around $1 per hour, according to Shah, compared to up to $90 per hour for a nurse, also enables hospitals and other health care organizations to afford to offer these services to more people.
“What are the things we don’t even do today that we would do [with an LLM]? Would we call every patient two days after they start every medication just to check in and see if they’re having any weird side effects? Of course we would,” says Shah.
“Would we give every single person a chronic care nurse that has two or more chronic diseases, which is around 68 million people? We don’t have 68 million people’s worth of nurses. We have 3 million [registered] nurses in the whole country. They couldn’t possibly handle 68 million people on a chronic care basis, calling them every week. It wouldn’t work. But the AI can.”
The way in which generative AI can complete these tasks can often seem like magic, but ultimately it comes down to a system’s ability to make predictions about which words should follow the next — somewhat like an extremely advanced autocomplete program. But unlike autocomplete, LLMs can learn to generate long-form, complex responses by incorporating conceptual knowledge and referring to previously generated text for context.
Gaining the right knowledge requires training the model on the right dataset, and Hippocratic AI’s team includes both AI researchers and health care experts to do this training. The idea is to create a model that consistently produces responses that real practitioners would approve of. As these responses accumulate, the model improves. The company’s LLM is learning quickly; it has already surpassed GPT-4 in 105 out of 114 health care examinations and certifications. With a dedicated focus on health care applications, the company’s LLM is more equipped to handle the nuance of the required responses than an all-purpose model like GPT. Shah is relying on experienced medical professionals to help build out this expertise.
“We put them on our safety council. We say, help us test the model. Nurses who do the chronic care calls today are the best ones to judge whether our algorithm is good at doing chronic care calls,” he says.
“Let’s have them judge the product on a daily basis, on a weekly basis. Then we work with them on the use cases that are the safe ones to choose, the ones that they’re willing to deploy. Then you build a product, but now you’ve built the product together with them in collaboration, and you just end up with a much safer, better product that’s more tuned to your customer.”
In the end, it may be nurses who help tune LLMs to act as a stopgap to the staff shortages that have so drastically altered their field in recent years.
Have you read?
Report: Grenada Citizenship by Investment Programme, 2023.
Report: Jordan Citizenship by Investment Programme, 2023.
Report: Malta Citizenship by Investment Programme, 2023.
Report: Saint Lucia Citizenship by Investment Programme, 2023.
Report: St Kitts and Nevis Citizenship by Investment Programme, 2023.
Add CEOWORLD magazine to your Google News feed.
Follow CEOWORLD magazine headlines on: Google News, LinkedIn, Twitter, and Facebook.
Copyright 2024 The CEOWORLD magazine. All rights reserved. This material (and any extract from it) must not be copied, redistributed or placed on any website, without CEOWORLD magazine' prior written consent. For media queries, please contact: info@ceoworld.biz