Why Every CEO Needs to Understand Data Science
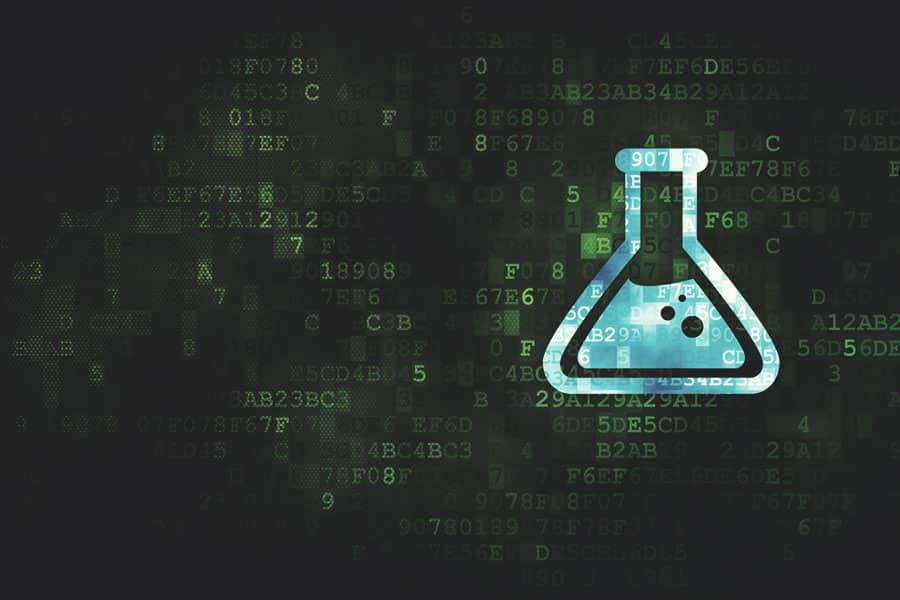
Tech culture has perpetuated the myth that data science is a sort of magic; something that only those with exceptional math skills, deep technical know-how, and industry knowledge can understand or act on. While it’s true that math skills and technical knowledge are required to effectively extract insights from data, it’s far from magic. Given a little time and effort, anyone can become familiar with the basic concepts.
As a CEO, you don’t need to understand every technical detail, but it’s very important to have a good grasp of the entire process behind extracting useful insights from your data. Here are five big-picture steps you must take to ensure you understand data science (and to ensure your company is gaining actionable insights throughout the process):
1) Become a partner. Data science experts often have very narrow, specialized-knowledge silos, which makes them well-suited for gathering and manipulating data. However, without direction from someone with wider business knowledge, it can be difficult to know what to focus on.
It’s important to partner with your data scientists and give them direction without being overbearing. Data science is still somewhat of an art form, and while direction is useful, data scientists still appreciate a level of artistic freedom to get creative. This creativity will often pay dividends via new or novel analysis and should be encouraged as long as useful results are being achieved.
2) Understand big data is not data science. It’s important to differentiate between big data and data science because the two are often confused. While big data can be part of a data science project, it’s still relatively unusual to use true big data in actual projects. Data that is scattered among many data sets and sources is a much more common — and often more challenging — real-world scenario.
A good data scientist has the technical skill to pull all of these sources together and bring the information into a single data stream so each data set can be combined with others. Combining data from multiple sources is often the best way to gain insights, and the results can be surprising, especially when two data sets seem unrelated prior to further analysis.
3) Leverage industry best practices. As data science develops, various best practices have emerged. Some of these practices are universal, and some are industry-specific. Pay attention to your competitors, and learn from their successes and failures.
Consider joining an industry-specific data science group, or create one if there are no groups for your industry. As a result, competitors will be able to collaborate in a neutral environment to the benefit of all parties.
While sharing data insights with your competitors may seem like a disadvantage, it can often be truly beneficial: Gathering insights from your data is not a zero-sum game. You may discover advantages or insights that are unique to your business by running a competitor’s model on your own data.
This is similar to the open sourcing of key infrastructure components undertaken by tech companies like Netflix and Facebook. While they are ostensibly providing tools to their competitors, the collaboration and contributions of their competitors have been net positives for the company.
4) Think critically. Once your data has been gathered into a single source and your tools are in place for manipulating and visualizing the data, it’s time to start working on models. It’s important at this stage to apply critical thinking in the development of your model.
Data tools and industry knowledge are fantastic, but without a level of critical thinking and mathematical modeling, it’s very difficult to extract useful insights. This is why it’s important to work alongside your data scientists and ensure they have the industry- and domain-specific knowledge they need to succeed.
5) Acknowledge that a ‘one size fits all’ tool doesn’t exist. Data science teams are comprised of individuals with different academic backgrounds and experiences. Each plays a critical role in acquiring, cleansing, and exploring the data to build predictive models using various data science techniques.
Given the diversity of the data science team, there needs to be more than one way to empower the data science team.
Getting Started With the Right Tools
Making sure the people closest to the data have the right tools is critical to success for any data science project. As you roll out your big data initiatives, be sure to keep realistic expectations for each project. While you should absolutely track the return on investment for each project, the timeline used may need to be a bit longer than other engineering endeavors.
Depending on how your data is currently organized and obtained, just collecting and consolidating your data into a useable source and format can be a herculean task. Generally, you should not expect any useful analysis until after your data is identified, cleaned, and converted to computationally efficient formats.
Fortunately, you don’t need to commit to a full data science team right away. Dip your toes in the water, and see what it’s like to use data to produce an actionable result for your business. Third-party vendors can often help you get started with a proof of concept that you can then develop further with in-house talent.
It’s incredibly beneficial to start small and work up to a larger team as your data practices and experiences within the company become more established. Because once you have created best practices for your data collection and retention pipelines, it’s much easier to onboard new data scientists and technical staff. You’ll also be able to adapt to different data science challenges along the way, such as deciding whether to mine existing data, enrich current data with new information, or work with new data altogether for different projects.
Every modern business should have ongoing data science initiatives. If you aren’t utilizing your data, you can be sure your competitors are, and without a data program of your own, you risk being left behind by the competition. Keep these points in mind to ensure your data science rollout goes smoothly and delivers maximum impact to the bottom line (without thinking of it as simply magic).
+++++++++++
Have you missed?
Meet The World’s Top 20 Smartest Tech Investors, 2016
Strengthen your brand in a recession
5 qualifications to look for in every cybersecurity executive
The Future is Now: Virtual Reality Creates New Branding Opportunities
++++++++++
By Travis Oliphant, Co-Founder & Chief Executive Officer of Continuum Analytics.
Bring the best of the CEOWORLD magazine's global journalism to audiences in the United States and around the world. - Add CEOWORLD magazine to your Google News feed.
Follow CEOWORLD magazine headlines on: Google News, LinkedIn, Twitter, and Facebook.
Copyright 2025 The CEOWORLD magazine. All rights reserved. This material (and any extract from it) must not be copied, redistributed or placed on any website, without CEOWORLD magazine' prior written consent. For media queries, please contact: info@ceoworld.biz